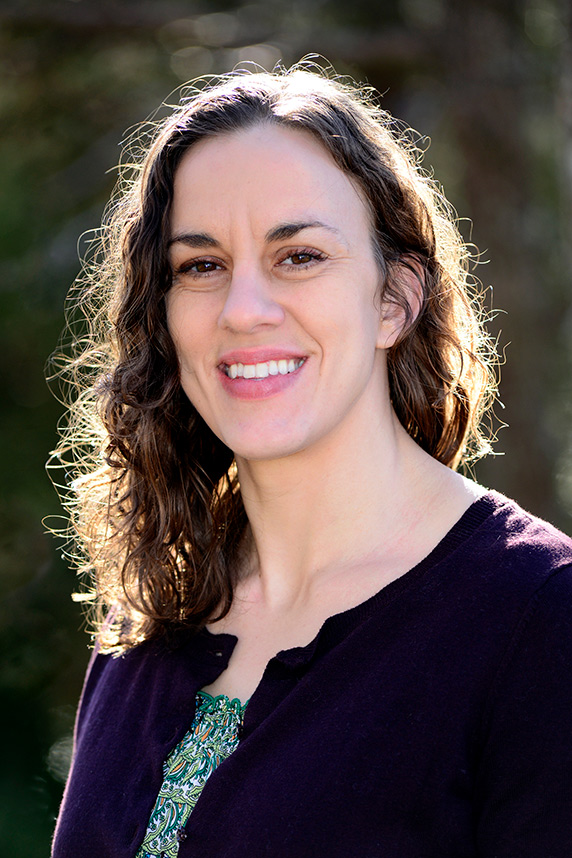
Scientists who study how chemicals and the environment may affect our health must grapple with the fact that real-world exposures involve mixtures. An individual’s everyday experience involves an array of substances from many sources, such as consumer products, diet, and occupational settings. Consider traffic pollution, which alone contains volatile organic compounds, particulate matter, nitrogen oxides, and other agents.
To understand how mixtures may cause harm, researchers need to study the effects of individual agents and how the substances interact, as well as assessing the combined effects. Increasingly, scientists also need to analyze large datasets that include complex variables, diverse study populations, and even socioeconomic factors. NIEHS grant recipients participating in the institute’s Powering Research Through Innovative Methods for Mixtures in Epidemiology (PRIME) program met online Oct. 14 to address such challenges and share their work.
“The goals of PRIME are to enable novel statistical methods for mixtures, to compare the approaches across newly discovered and existing ones, to facilitate development of statistical software, and to provide resources for education and cross-training,” said Bonnie Joubert, Ph.D., a health scientist administrator in the NIEHS Population Health Branch (PHB), who directs PRIME. She and Toccara Chamberlain, a PHB health specialist, helped to organize the event, the second such meeting for the program, which began in 2017 (see sidebar).
Adjusting for study bias
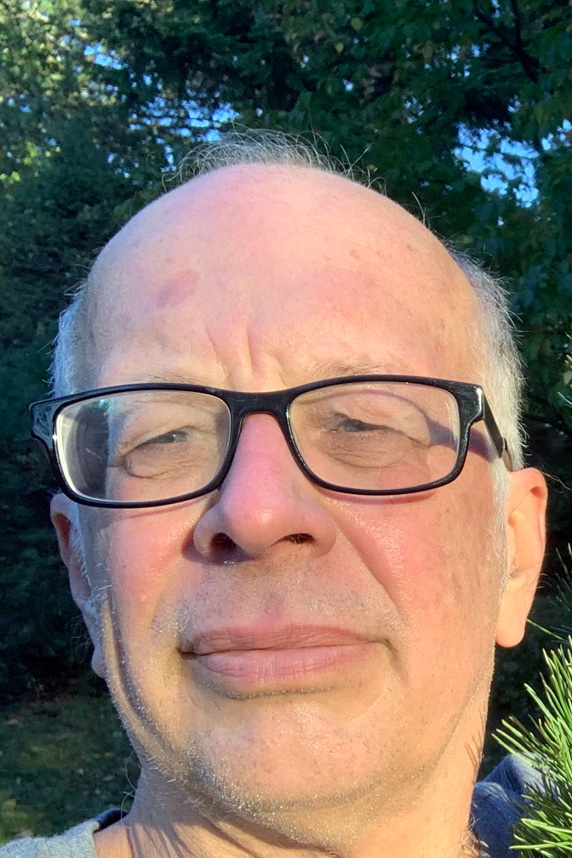
PRIME grantees, their collaborators, and trainees tackled big scientific problems through a quantitative lens. For example, in studying mixtures, researchers hope to show whether there are associations between a given exposure and a specific biological change or health outcome. A key task involves adjusting for confounders, which are variables that can bias associations.
“The moral of the story for mixtures epidemiology is that if you blindly include multiple exposures in the same model, you can get into the problem of incorrectly interpreting all results as causal estimates,” said grantee Thomas Webster, D.Sc., from Boston University. “You need to take into account the difference between looking at estimates for each individual exposure in the model and the overall effect of the mixture, which is a unique aspect of this kind of research.”
Webster pointed to biomarkers of exposure, which show measurements of chemicals and their metabolites in the body. He said that such biomarkers are used widely in mixtures epidemiology because they are relatively inexpensive and more accurate than attempting to assess exposure levels from external sources, such as air samples. However, these biomarkers can be susceptible to confounding involving individuals’ behavior and physiology, according to Webster.
Risk assessment, COVID-19, and facial recognition tech
Below are some other presentations from the PRIME meeting, which also included four-minute lightning talks by 16 early-career scientists and a panel discussion on various data challenges.
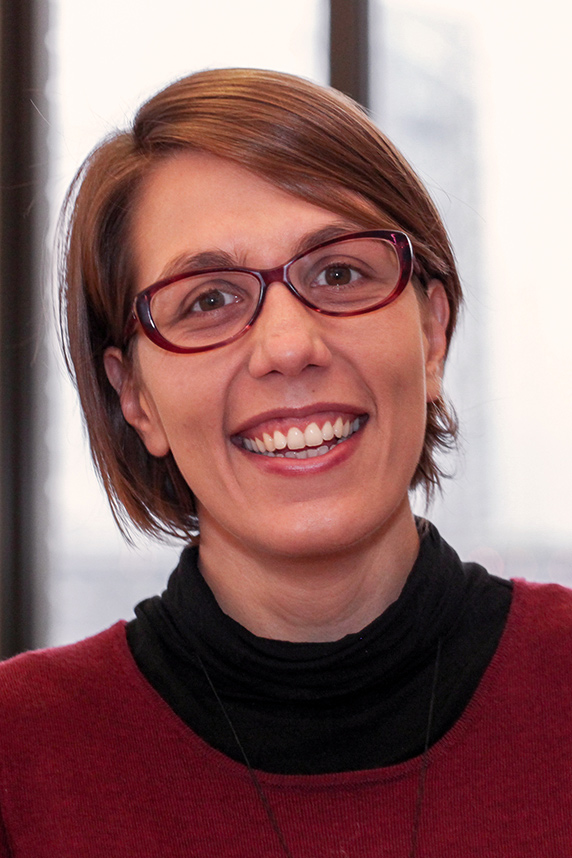
- “Bringing Modern Data Science Tools to Bear on Environmental Mixtures.” Marie Lynn Miranda, Ph.D., from the University of Notre Dame; Kathy Ensor, Ph.D., and Daniel Kowal, Ph.D., both from Rice University.
- “Methods for Data Integration and Risk Assessment for Environmental Mixtures.” Brent Coull, Ph.D., from Harvard University, and Chris Gennings, Ph.D., from the Icahn School of Medicine at Mount Sinai.
- “Transformative Statistical Toolbox for Epidemiologists Studying COVID-19.” David Dunson, Ph.D., from Duke University.
Marianthi-Anna Kioumourtzoglou, Sc.D., from Columbia University, discussed her work using a method called principal component pursuit — which is used in face recognition and medical imaging applications — to enhance exposure pattern recognition.
“If we can identify specific sources of exposure or behavior patterns in a study population and subsequently link these to adverse health outcomes, we can use this information to inform policy,” she said.
Promoting collaborative science
“This program is really a great example of the promotion of collaborative science,” noted NIEHS Acting Deputy Director Gwen Collman, Ph.D.
She explained that efforts by PRIME grantees align well with the NIEHS Strategic Plan 2018-2023. Collman cited theme two of the plan, “Promoting Translation — Data to Knowledge to Action,” as an example of such overlap.
“We want to explore datasets of a variety of sizes and shapes to create actionable knowledge that will be useful both to scientists and community members, to prevent and protect ourselves from exposures,” she said.
Joubert noted that PRIME grantees now use GitHub to share their datasets and statistical software code, and she said that more material will be added to the site over time.