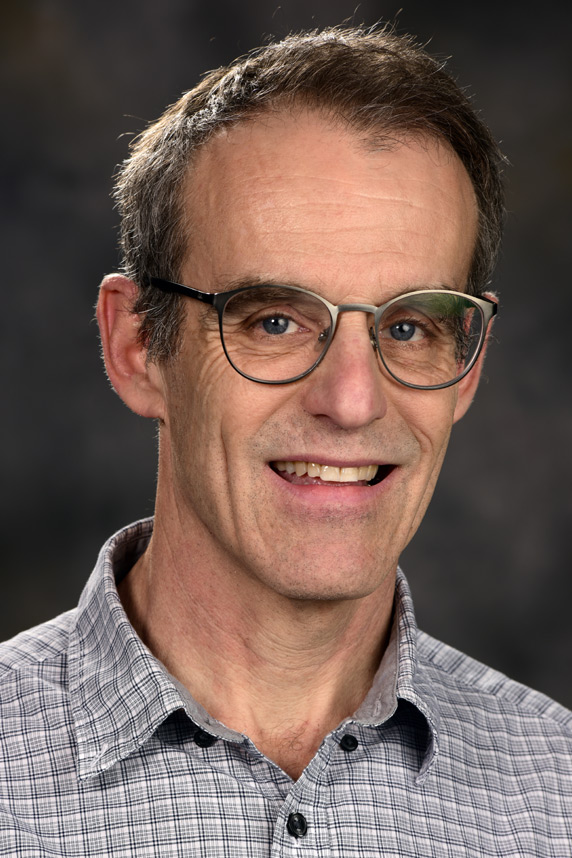
Scientists have been generating and using synthetic data for more than 60 years, according to David Resnik, J.D., Ph.D., a bioethicist at NIEHS. This mock data mimics real-world data but does not come from actual measurements or observations. With rapid advances in machine learning and generative artificial intelligence (GenAI) systems, such as ChatGPT, the use of synthetic data in research has grown.
Exploring the ethical implications tied to the rise in GenAI synthetic data is the focus of an opinion piece Resnik and co-authors published in the Proceedings of the National Academy of Sciences, March 4. Environmental Factor recently spoke with Resnik about what makes synthetic data generated by AI different, challenges scientists now face, and steps the scientific community can take to safeguard research data.
Environmental Factor (EF): How can synthetic data benefit environmental health research?
David Resnik: One valuable use of synthetic data — whether produced by GenAI or another process — is for modeling phenomena. Let’s say you have a hypothesis or theory in environmental science — you can generate some synthetic data to test it before conducting a real field study. This model can tell you things about the testing process and determine whether it’s worth pursuing.
Another potential use, though not common at NIEHS, is creating a digital twin of a person. This is a model that mimics a person’s data — like their height, weight, and other characteristics — but doesn’t include enough details to identify them. This allows for sharing data without privacy concerns. Digital twins can be useful for modeling, hypothesis testing, and developing theories.
EF: What made you want to write this opinion piece now?
Resnik: The potential for research misconduct, combined with the ability of generative AI [GenAI] to create highly realistic fake data, makes for a ticking time bomb. We could see lots of fake data infiltrating the scientific community, if we're not proactively trying to stop it.
While research misconduct is rare, with some estimates saying it affects only about 1-2% of research, even that small rate is extremely destructive and can harm the trust people have in the scientific process and the institutions involved.
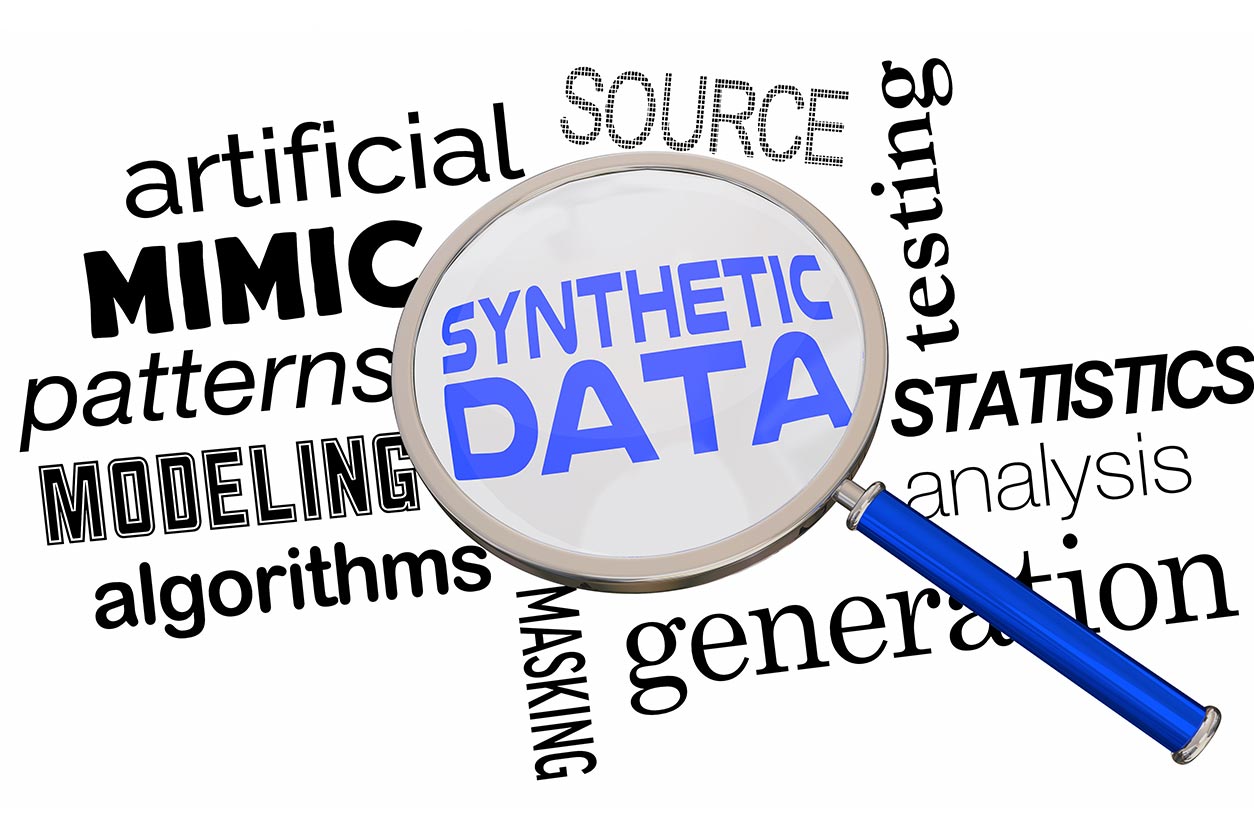
EF: What concerns you about synthetic data generated by AI?
Resnik: I have two main concerns. The first is accidental misuse, where synthetic GenAI data is mistakenly treated as real data, which could corrupt the research record. One way to deal with that is to watermark synthetic GenAI data so that everyone knows what it is and doesn’t treat it as real data. However, that might not be enough. Although most journals and databases clearly mark retracted papers, scientists often continue to cite them long after they’ve been retracted, either because they don’t notice the retractions or ignore them.
The second concern is the potential for deliberate misuse, where people intentionally fabricate or falsify data, passing it off as real without revealing that it’s synthetic. That’s a much harder problem to deal with. We have tools to detect plagiarism, AI-generated writing, and AI-generated images. But detection is getting harder. There’s essentially a race unfolding between computer scientists developing systems to detect synthetic GenAI data and those developing ways to evade these tools.
EF: How else can scientists address the ethical challenges of synthetic GenAI data?
Resnik: The ultimate thing we have to keep in mind is that no technical solution is ever going to be perfect. I think it would be helpful if journals, funding agencies, or academic institutions developed some guidelines, starting with a basic definition of synthetic data and its acceptable uses. And we could always ask scientists to sign an honor code certifying that all the data they are publishing is real.
But the only thing we really have to fall back on is ethics. We just have to teach people to do the right thing, even when this technology is available to them.
NIEHS has maintained a strong commitment to training people in the responsible conduct of research, which is necessary to protect the integrity of research data today and in the future.
(Marla Broadfoot, Ph.D., is a contract writer for the NIEHS Office of Communications and Public Liaison.)